Call :
AI Call 2020
Project status :
Completed
Key application areas


Countries involved
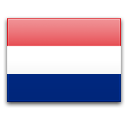
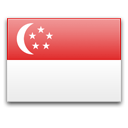
Project leader(s)
Koen SmitKey project dates
March 2021 - February 2024The AQUA project aims to develop machine learning frameworks capable of correlating data from water quality and process monitoring sensors at both local and network levels. [AQUA]
Fresh water is scarce, with only 2.5% of global water potable, making reliable water quality monitoring crucial. Current systems fail to classify contaminants effectively, cosing risks to public health. This project aims to develop advanced sensor networks and machine learning tools to create a digital twin of water networks, enabling real-time, accurate monitoring and management of water quality.
Achievements and results of the project
Data from broad-spectrum water quality sensors (EventLab, AquaTEC) is fused with additional sensor data and enhanced by AI/ML for event detection and classification at local node level. Furthermore, a data model ontology is created to support detailed network descriptions, enabling effective data organization and analysis. Additionally, a central platform for data acquisition and processing is developed integrating Artificial Intelligence (AI)/Machine Learning (ML) tools for both local and network-level water quality event detection, classification, and traceability. This platform is demonstrated in a drinking water distribution network.
Background, objectives of the project and challenges
Because only a small portion of the Earth’s water is considered consumable, the demand for reliable water quality monitoring is critical. Environmental changes, urbanization, and industrial activities increasingly threaten fresh water sources, complicating efforts to ensure safe and sustainable water supplies. Conventional water monitoring methods often rely on
manual sampling or simple sensors that provide limited, periodic data. Such methods are often insufficient for detecting and responding to the full spectrum of potential contaminants in real time, posing risks to public health and ecosystems. The primary challenges in water quality monitoring involve data collection, aggregation and scaling, and rapid responsiveness. Water networks require a system that can process vast amounts of data from different types of sensors. Many existing systems face compatibility issues, as various sensors operate
differently. Achieving both local-node and networklevel accuracy in such a setup presents a significant technical challenge. Moreover, traditional data processing models struggle with real-time classification and traceability of contaminants, making it difficult to detect emerging threats or anomalous events promptly. Another challenge lies in balancing centralized data
with local processing capabilities to achieve efficient monitoring without sacrificing the precision needed for critical decision-making.
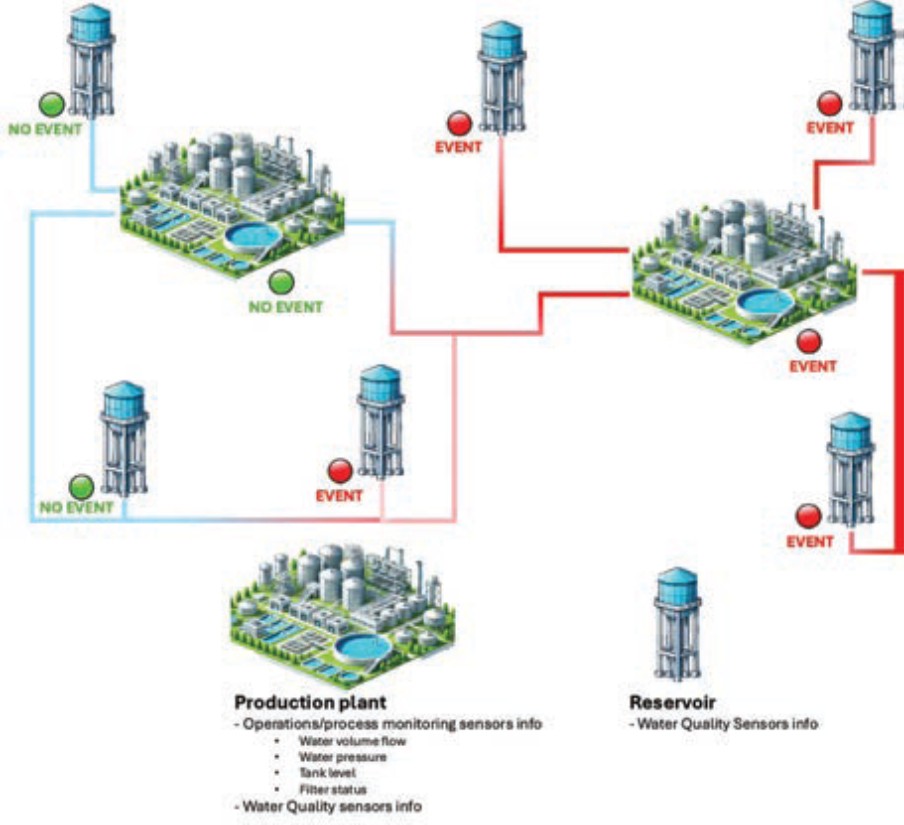
The AQUA project aims to establish an industry specific platform for democratizing data science & ML operations, enabling robust, continuous monitoring of water quality. By combining data from different water quality sensors with operational data into a single, adaptable system, the project seeks to detect and classify water quality events in real time, fostering a more proactive approach to water safety. This framework will also allow for the traceability of contaminants, improving the capacity to track sources and impacts across large water networks.
Technological achievements
The AQUA project has delivered several advancements in water quality monitoring, focusing on real-time data processing, anomaly detection, and enhanced sensor technologies.
A key achievement is the development of an all-inone cloud-based data science platform for the water industry. Build around a user-friendly dashboard, the platform integrates high data loads and real-time streams, facilitating seamless data flow and analysis across various water quality monitoring systems. A network-based anomaly detection model is integrated
that uses ML to identify and categorize anomalies within water distribution networks, providing early warnings and actionable insights for proactive responses. This data platform, enabled by Dimensions from HAL24K, uses data model ontology for water network data elaborated in this project, improving communication and data management and enhancing the application of AI and ML for more effective real-time monitoring and response capabilities. A complementary AQUA data platform is developed for local-node sensor data collection, processing and distribution. Combining sensor data, it uses a “fingerprinting” technique to classify water quality changes enabling water utilities to quickly identify and respond to contaminants. Finally, developments were made to local-node broadspectrum water quality monitoring systems EventLab and AquaTEC. EventLab is a next-generation monitoring
tool using refractive index for enhanced water quality event detection. The integration of additional sensors reduced false positives and ensures more accurate and robust event detection. AquaTEC is a system that uses advanced ML and computer vision to monitor fish behavior, providing deeper insights into the biological effects of water quality changes. The project enhanced systems performance by refining its ML algorithms and integrating additional sensors, improving its ability to detect abnormalities in fish behavior and correlate with
fluctuations in water conditions.
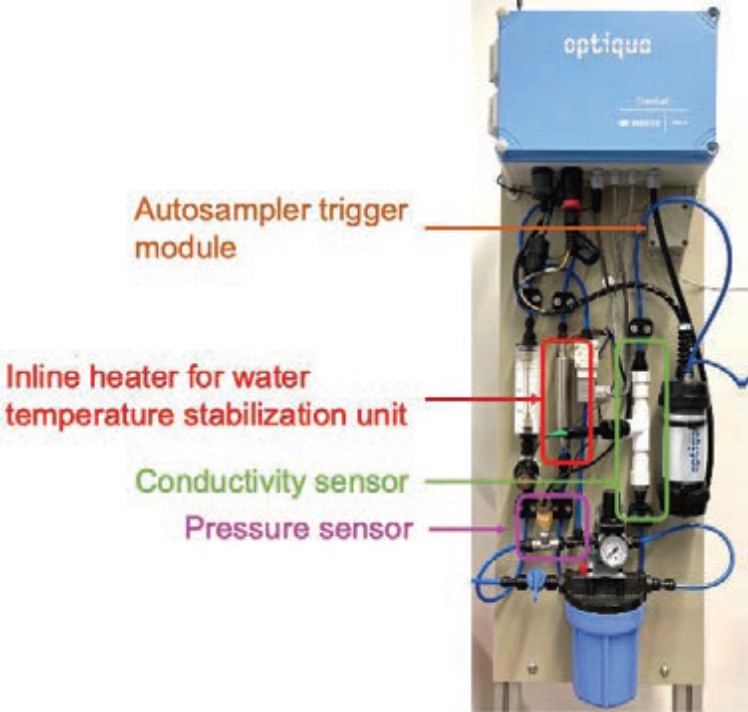
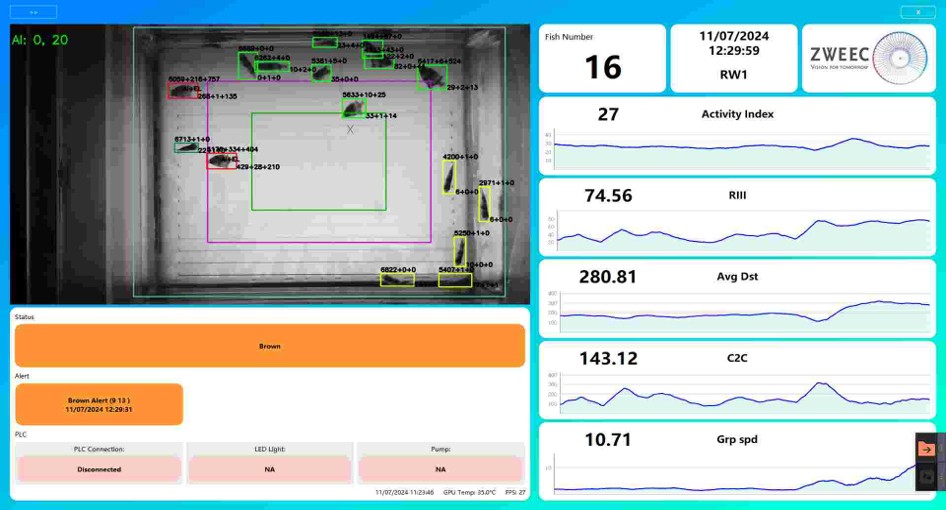
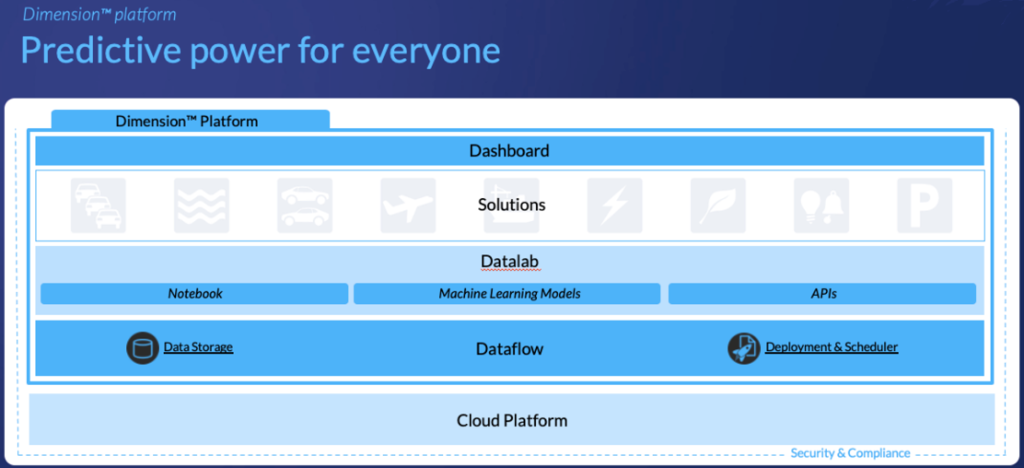
Market Potential
The innovations from the AQUA project have potential for market penetrationin the water sector, particularly in Europe and Asia-Pacific, where early collaborations and validations have
already been established within the consortium. When these connections are successfully leveraged, the consortium is well-positioned to expand into other regions. The project outcomes
offer scalable, cost-effective solutions tailored to market needs in localnode and network-level water quality monitoring. Despite the conservative nature of the water industry, the demonstrated AQUA-platform benefits create a solid foundation for gradual adoption and growth, especially in real-time monitoring scenarios. With the water quality monitoring market
currently valued at $5.35 million and expected to expand at a CAGR of 7.1% to 2030, the market potential provides opportunities for the consortium of the project. Prospects for the developed concepts in the broader utility sector are to be explored further.
Societal & Economic Impact
This project addresses critical water quality challenges across regions with diverse needs. By enhancing real-time monitoring and anomaly detection, it strengthens safeguards against emerging risks from climate change, industrial discharge, and growing population demands. These advancements enable water utilities to identify and mitigate quality changes more rapidly, ensuring safer drinking water. The technologies also support sustainable water management, aligning with global efforts to reduce water stress and improve operational resilience.
Patents, Standardisation, Publications
Three publications emerged from this project consisting of two BSc theses and one intern report from Saxion University of Applied Sciences. In June 2022: “Detecting Contamination in Drinking Water” by Darlaine Pardo and “Detecteren van verontreinigingen in drinkwater” by Nelienke Luijten. Additionally, in January 2023: “Identificatie van geselecteerde vervuilingen in stromend water met behulp van Machine Learning” by Tom Scheper, exploring contamination identification through machine learning techniques. It is yet to be decided if the consortium will publish the results. No patents were filed.
Future Developments
Optiqua will continue testing its Next Generation EventLab system and review its business case for bringing the system to production. Additionally, Zweec aims to improve AquaTEC by utilizing anomaly detection and enhancing correlation between network type of physical sensors and AquaTEC alerts. Furthermore, HAL24K will expand its anomaly detection model to other networks and develop a water demand model on the AQUA platform. Finally, De Watergroep will advance AQUA’s methodology and ontology, aligning with data processes and promoting international utility cooperation.