Project status :
Completed
Key application areas


Essential capabilities

PARTNER
Direct conversion AB / Ecole des Mines de Saint Etienne / Ericsson AB / Excillum AB / Fraunhofer IMWS / Gimic AB / Infineon Technologies AG / Jean Monnet University (Saint-Etienne) in Lyon University / Kern Microtechnik GmbH / Materiex AB / Matworks GmbH / Orsay Physics / PVA TePla Analytical Systems GmbH / RISE / Robert Bosch GmbH / STMicroelectronics (Rousset) SAS / STMicroelectronics (TOURS) SAS / STMicroelectronics Grenoble 2 SAS / TESCAN ORSAY HOLDING / University of Stuttgart / cyberTECHNOLOGIES
Direct conversion AB / Ecole des Mines de Saint Etienne / Ericsson AB / Excillum AB / Fraunhofer IMWS / Gimic AB / Infineon Technologies AG / Jean Monnet University (Saint-Etienne) in Lyon University / Kern Microtechnik GmbH / Materiex AB / Matworks GmbH / Orsay Physics / PVA TePla Analytical Systems GmbH / RISE / Robert Bosch GmbH / STMicroelectronics (Rousset) SAS / STMicroelectronics (TOURS) SAS / STMicroelectronics Grenoble 2 SAS / TESCAN ORSAY HOLDING / University of Stuttgart / cyberTECHNOLOGIES
Countries involved
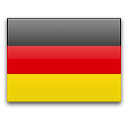
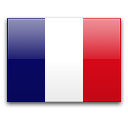
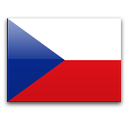
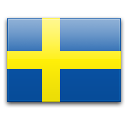
Project leader(s)
Klaus PresselKey project dates
01 April 2020 - 30 September 2023Failure Analysis 4.0 – Key for reliable electronic devices in smart mobility and industrial production [FA4.0]
FA4.0 introduced digitalization into innovative failure analysis (FA) methods and equipment to ensure that increasingly complex electronic systems operate with high reliability and safety in daily use.
In FA4.0, 4 semiconductor and system suppliers, 11 equipment suppliers supported by 4 RTOs combined their strength to develop innovative FA methods and equipment to tackle challenges of heterogeneous integration and miniaturization in microelectronics. For the first time new methodologies based on artificial intelligence (AI), machine learning (ML) and digital twin technologies were applied to improve the FA equipment and methodology landscape and provide highly efficient workflows for failure root cause analysis
Achievements and results of the project
The results of FA4.0 demonstrated impressive improvements in AI enhanced failure analysis. It was demonstrated that enabling and implementation of AI methodologies and advanced data analysis including digital twin technologies have deep impact on the efficiency of FA. Advanced imaging and signal analysis algorithms including neuronal network algorithms and correlative data analysis were developed to push the performance of FA tools (see Figures 1a and b). The ML based image analysis (Figure 1b) achieved a 5 times faster analysis and more than 90% accuracy improvement compared to existing solutions. Several FA equipment have been enhanced by improving hardware components and novel AI algorithms to achieve higher sensitivities and resolution. Furthermore, standardized data-exchange and an innovative universal sample holder were implemented to combine equipment to highly efficient and more automated workflows.
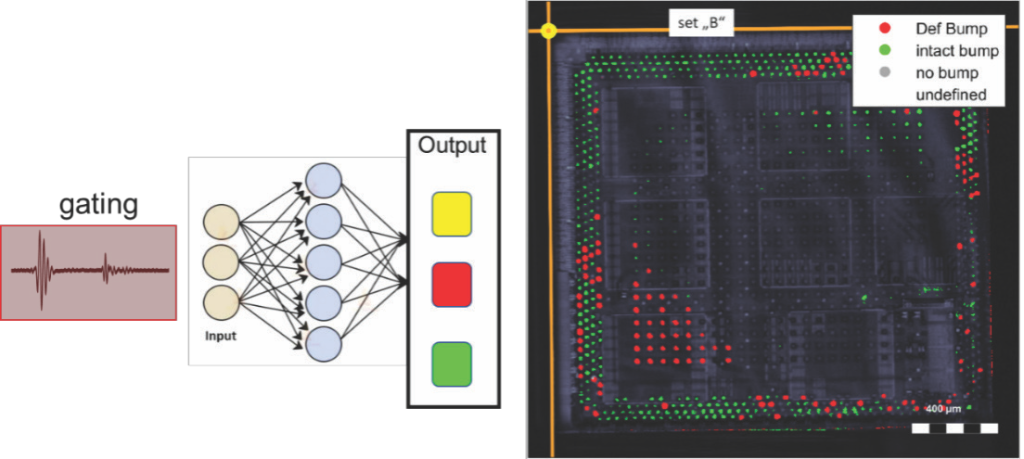
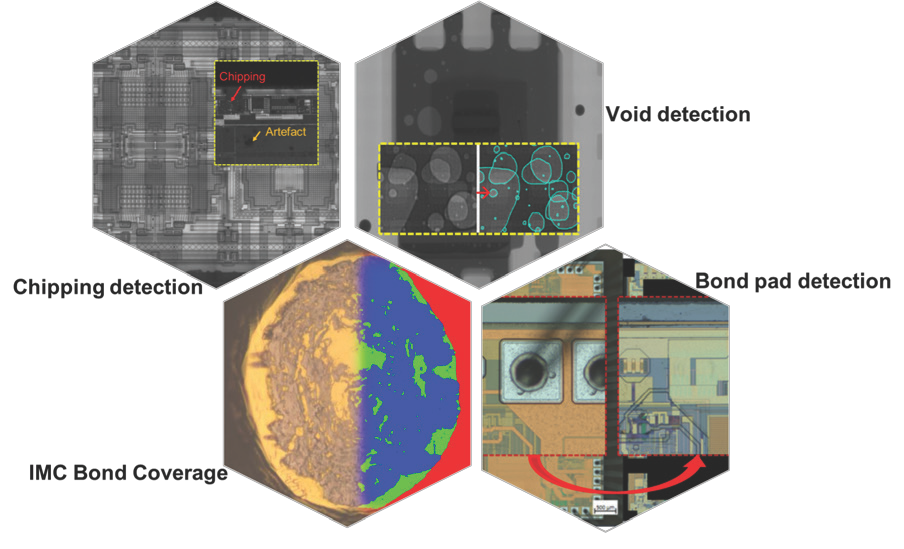
Results from each use-case were presented at IEEE CASE
2021, CAM-Workshop 2022 and 2024 and AIMSE 2023.
Background, objectives of the project and challenges
Miniaturization and heterogeneous integration of more functionality in smaller volume drive the development of more and more compact and complicated microelectronic systems. Semiconductor and system companies have an increasing effort to achieve reliability and quality. Effort and cost for FA increase typically by 3-5% per year. Thus, new methods and tools to improve performance, efficiency and speed are required to tackle this challenge.
Objective of the FA4.0 project was the development of innovative FA equipment and methods in specific applying new capabilities of computer and data science. This includes AI, ML and digital twin technologies in combination with automation of failure analysis tools and workflows.
The integration of newly developed AI based algorithms for image and signal analysis allowed the enhancement of tool performance. Failure analysis methodologies, which used the developed universal sample holder, allowed improvement of through-put by interlinking equipment from different vendors for efficient comprehensive workflows.
The results of FA4.0 demonstrated that it is possible to establish an infrastructure in order to exchange meta-data and other information between equipment of different tool suppliers. This is a basic requirement for improving the FA methodology and efficiency. The outstanding important step achieved in FA4.0 was the introduction of integrated workflows (combined equipment), including a JSON (Java Script Object Notation) meta-data header and a universal sample holder. A standardisation initiative has been started under the umbrella of SEMI.
Technological achievements
The main technological achievements of the FA4.0 project are in respect to
• Computer and data analysis:
Extended image and signal analysis techniques to include ML based approaches by novel AI methods for data handling (see example Figure 3), pre-processing, labelling and training and implementation into tool platforms for automated void detection (Gimic), automated delamination detection (PVA TePla), automated TEM lamella preparation (TESCAN/ORSAY). Impressive enhancement of tool performance was demonstrated e.g. for SAM or AI image data analysis of intermetallic compounds gold bond inspection with a 60x time reduction.
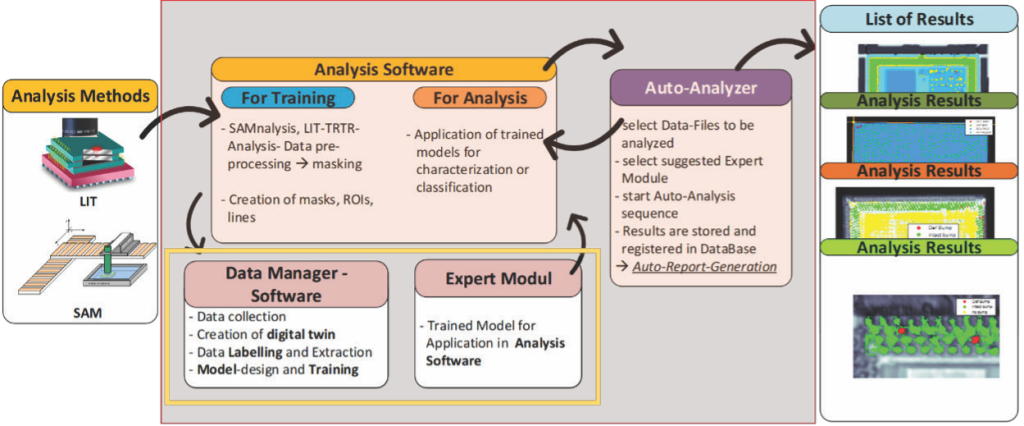
• Improved FA equipment:
— New X-Ray platform (see Figure 3) with novel X-Ray source (Excillum) improving the imaging resolution to 150 nm (3x improved), new detector concepts by Direct Conversion.
— New tool for thermal surface warpage measurements by CyberTECHNOLOGIES for wider T-range up to 300°C (see Figure 5).
— Enhanced FIB-laser preparation tools and workflows by ORSAY/TESCAN to reduce processing time (> 50 % time saving).
— Enhancement of scanning acoustic microscopy tool by PVA TePla with ML based automated defect detection and novel SAFT (synthetic aperture focusing technique) data analysis, improved data acquisition with a 20-30x time saving.
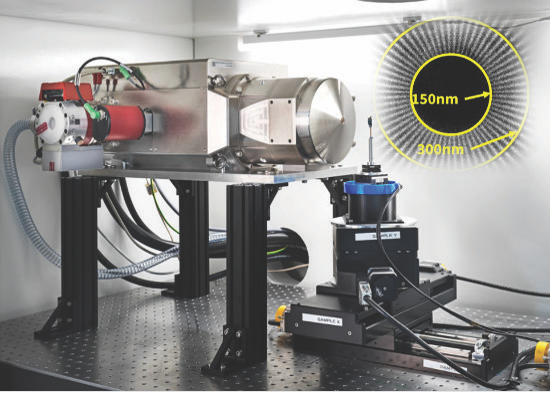
• Standardization
Standardized FA tool interfaces by a universal sample holder and harmonized data interface (JSON-header) which now can be applied to combine different FA equipment platforms to exchange meta-data and generate digital twins of samples (see Figure 4). In FA4.0 first applications of integrated workflows including tools of different vendors were demonstrated.
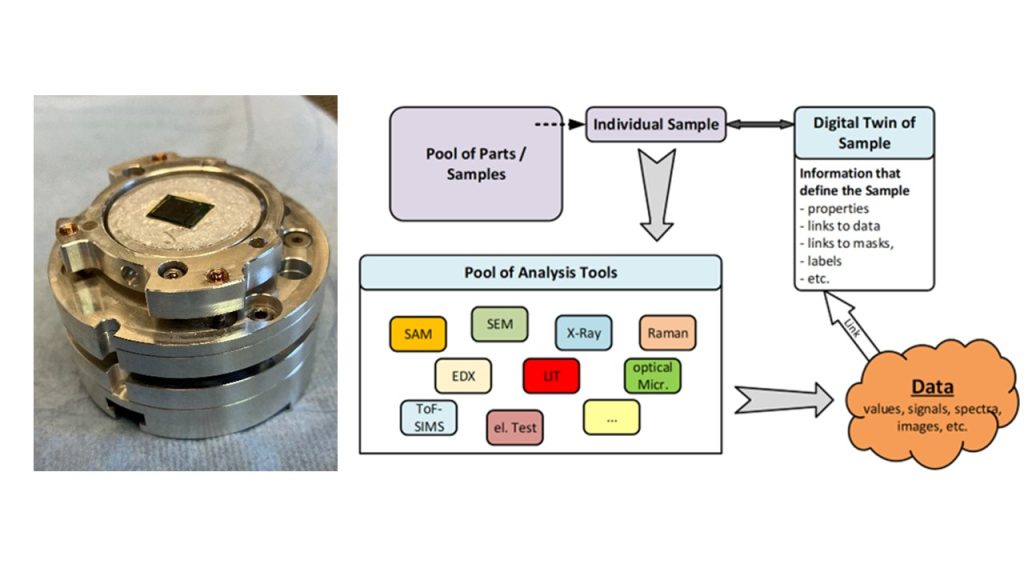
• Automated FA workflow
Several automated workflows for FA, enabling for the first time software-based defect navigation with higher accuracy and repeatability and automated end point detection for preparation were demonstrated.
Market Potential
The project opens new market potential for semiconductor and system suppliers, FA equipment suppliers, as well as software and design companies.
— Semiconductor/system suppliers are able to design, develop, and enter products faster into the market with better reliability and quality:
• Better access to European markets of high reliability and quality products like autonomous driving, energy etc.
• Better customer satisfaction by faster reaction on field returns.
— Failure analysis (FA) equipment suppliers are able to develop more efficient FA equipment (see e.g. Figure 5 on thermal warpage measurement) for faster FA support.
— Software companies like Matworks and Gimic get access to a new market both for equipment and processes.
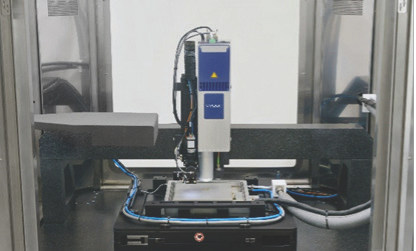
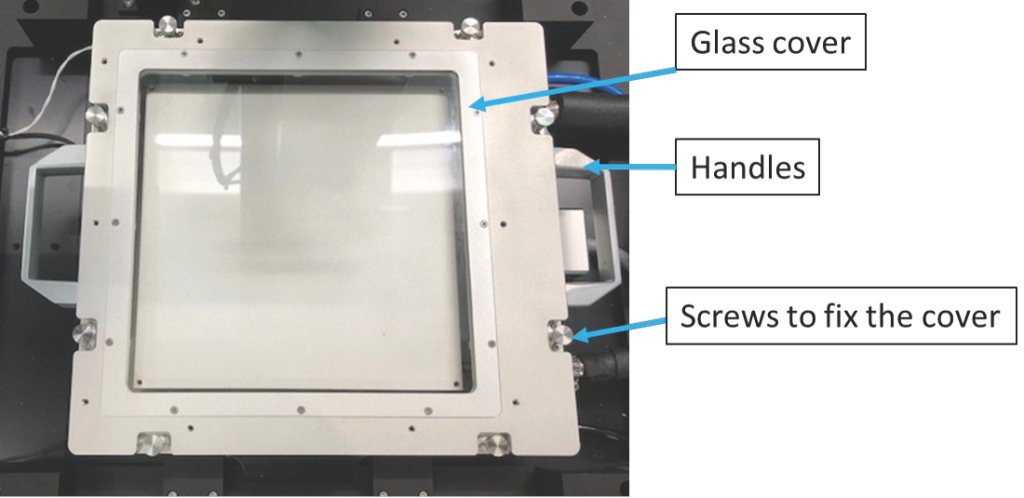
CyberTECHNOLOGIES for efficient FA
Societal & Economic Impact
Societal impact – new jobs
Three PhD and more than 10 master and bachelor theses were developed out of the project. Additional new job opportunities related to computer and data science arise due to the activities of the project (e.g. at Bosch, Infineon).
Environmental impact – Strengthening reliability which saves material
AI learning approaches support higher reliability and quality of products.
Economic impact by faster and more efficient failure analysis and standardization
AI enhanced analysis tools enable automated preparation and measurement routines saving time and personal effort. The analysis performance and speed can dramatically be improved (e.g. 20-30x faster SAM defect inspection, 60x reduced time for defect screening at intermetallic contacts).
Standardized FA tool interfaces and data handling e.g. by standardized unified sample holder and JSON data header, enable increasing speed of FA workflows and data analysis, reducing failure probability and thus increasing the overall efficiency of the failure analysis process (saving of hours, even days depending on workflow, the real potential is still a topic under investigation).
Patents, Standardisation, Publications
An international standardization initiative on FA tool interfaces started for the unified sample holder and JSON data header under the umbrella of SEMI. This standardization activity is supported by the international failure analysis conferences ISTFA and CAM-Workshop. The FA4.0 team organized special sessions at ISTFA 2022 “Standardized and AI Enhanced FA workflows” and the CAM-Workshop 2022, 2023, and 2024 to discuss AI and standardization topics.
A multitude of presentations and publications developed out of the project including a best poster award during IPFA 2022 at Singapore and best attendee paper at Singapore and a best attendee paper at ISTFA 2023 in Phoenix.
Several keynote presentations came out of FA4.0: Among them were the FA conferences IPFA 2022 at Singapore (“FA4.0-Exploring the Digital Future”), the CAM-Workshop (“Progress in Failure Analysis – A Key for Reliable High-quality European Electronics”), the NordPac Packaging Conferences (“Failure Analysis 4.0”) as well as the computer science conference AIMSE 2023 (“Impact of AI on Failure Analysis in Microelectronics”).
Future Developments
By the project, a new development trend was generated bringing together data science, AI and failure analysis experts. In summary “revolutionary” opportunities for failure analysis have been catalyzed in respect to automatization and performance of FA techniques and workflows.
FA4.0 is a door opener for future R&D: For example, the research in FA4.0 demonstrated that in a next step we need to push knowledge in data management e.g. set-up an ontology in failure analysis (see FA²IR project).
The following new projects, which are under preparation, came out of FA4.0:
— The FA²IR project with focuses on more digitalization and AI enhanced FA workflows already started meanwhile. This project also includes ideas to develop generalized AI models for different FA-related use-cases with data from different partners.— A large initiative in the Chips JU is under preparation that includes a network of FA users, equipment suppliers and an academy.
— A large initiative in the Chips JU is under preparation that includes a network of FA users, equipment suppliers and academy.